In this white paper, we propose an approach to sharing data sets for AI training as a public good governed as a commons. By adhering to the six principles of commons-based governance, data sets can be managed in a way that generates public value while making shared resources resilient to extraction or capture by commercial interests.
The purpose of defining these principles is two-fold:
We propose these principles as input into policy debates on data and AI governance. A commons-based approach can be introduced through regulatory means, funding and procurement rules, statements of principles, or data sharing frameworks. Secondly, these principles can also serve as a blueprint for the design of data sets that are governed and shared as a commons. To this end, we also provide practical examples of how these principles are being brought to life. Projects like Big Science or Common Voice have demonstrated that commons-based data sets can be successfully built.
These principles, tailored for the governance of AI data sets, are built on our previous work on Data Commons Primer. They are also the outcome of our research into the governance of AI datasets, including the AI_Commons case study. Finally, they are based on ongoing efforts to define how AI systems can be shared and made open, in which we have been participating – including the OSI-led process to define open-source AI systems, and the DPGA Community of Practice exploring AI systems as Digital Public Goods.
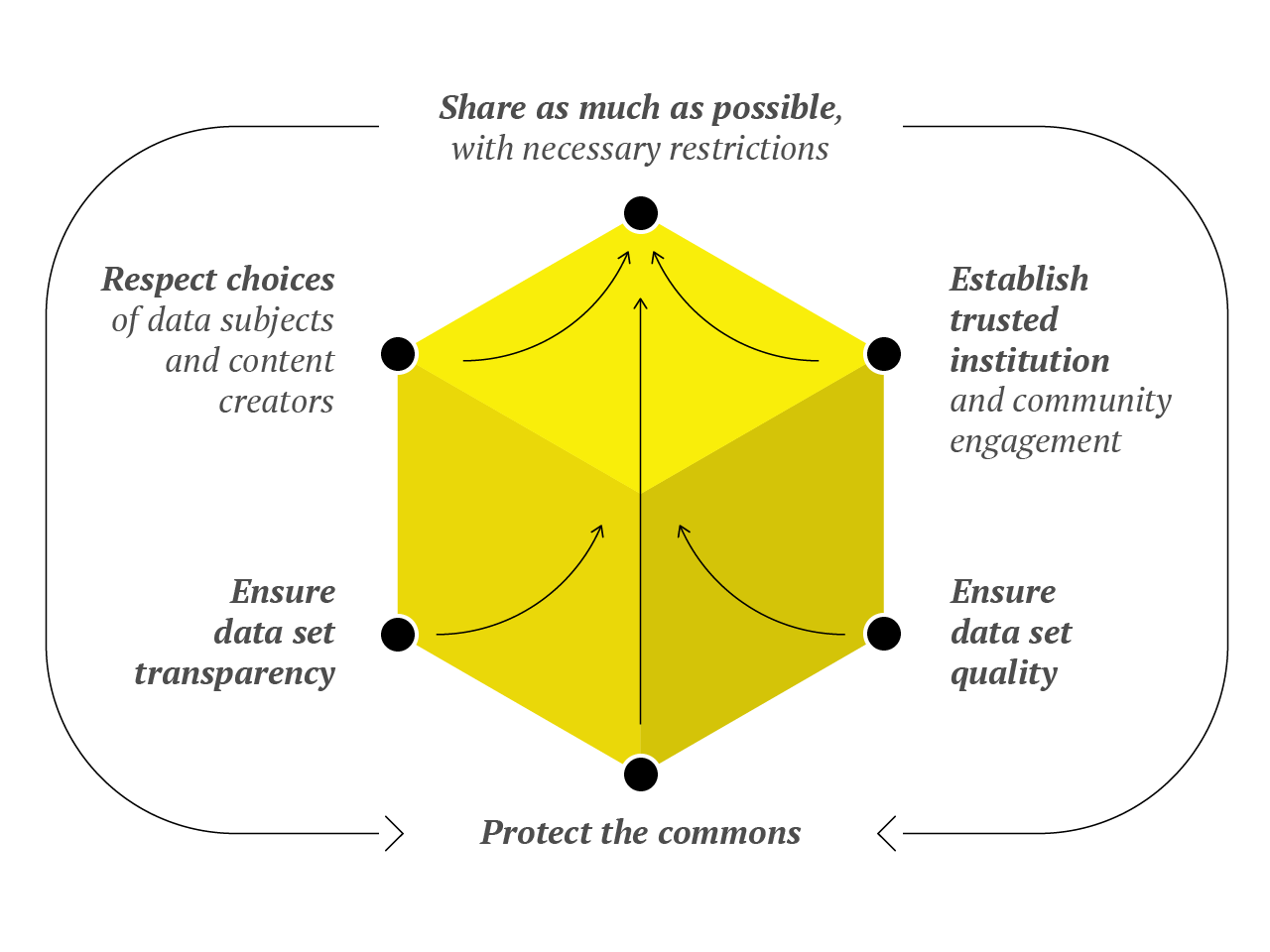
The six principles for commons-based data set governance are as follows:
- Share as much as possible while maintaining necessary restrictions: The first principle emphasizes the importance of defining essential restrictions on openness to protect various interests. It is critical to assess what aspects would benefit from openness and what challenges might arise. The governance of a data set requires a balance between open access and necessary restrictions, often implemented through open licensing and other access control measures.
- Be transparent about the data and provide documentation: Transparency about data sets, including thorough documentation of data sources and creation processes, is essential for informed discussions about AI and accountability in AI development. Standardized approaches to transparency, such as datasheets and data nutrition labels, facilitate information sharing and collaborative efforts to improve data sets. Without data transparency, it is difficult to monitor if shared resources are used in AI training.
- Respect the choices of data subjects and content creators: Data governance should respect the decisions of individuals who contribute data or creative works. Legal frameworks and voluntary measures help to ensure that the decisions of data subjects and content creators are respected, striking a balance between openness and individual agency.
- Protect the commons: Commons-based data set governance recognizes data sets as collectively owned and managed resources that serve both the community and the public interest. Mechanisms to protect the commons include consideration of working conditions, fair compensation, and mechanisms to ensure that value generated by the commons is returned.
- Ensure data set quality: Data set quality is critical to maintaining them as reliable and inclusive resources. Attention to data set quality includes addressing bias, ensuring data sets are free of discriminatory elements, distinguishing between human-generated and synthetic content, and creating purpose-built data sets to mitigate risks associated with publicly available data.
- Establish trusted institutions and ensure community engagement: Trusted institutions perform stewardship functions in the governance of commons-based data sets, ensuring proper management, access control, and fair treatment of contributors. Community engagement is essential for participatory governance, with the identification of relevant communities critical for democratic oversight and decision-making processes.
These principles are inspired by and rooted in existing best practices in AI data stewardship. For each principle, we provide examples of such practices. By publishing these principles, we hope to consolidate them and foster a dialogue with current and future dataset stewards and AI developers about how to implement them effectively and comprehensively. Together, we will look into how key datasets and data repositories align with and operationalize these principles. This process is essential to better understand the current landscape and guide future data governance decisions.
Read the paper